
Advance in EM
AI Beyond Human Eyes
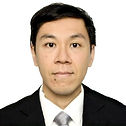
Dr KB Wong
Council Member, HKSEMS
A systematic review of diagnostic errors in the emergency department (ED) found that with estimated rates for diagnostic error (5.7%), misdiagnosis-related harms (2.0%), and serious misdiagnosis-related harms (0.3%) in EM setting. (1)
An estimated 795,000 Americans become permanently disabled or die annually across care settings because dangerous diseases are misdiagnosed (2). Medical errors, including healthcare-related adverse events, occur in 8–12% of hospitalisations in Europe (3). Common types of medical errors typically include surgical, diagnostic, medication, devices and equipment, systems failures, infections, falls, and healthcare technology (4). Medical errors are described as the third leading cause of death in the US (5). The prevalence and burden of missed diagnoses are significant, and this article will provide a brief overview of advancements in AI that see beyond our human capabilities.
AI includes machine learning, which encompasses a subset called artificial neural networks (ANNs). These networks mimic how biological neurons in the brain signal one another. The network architecture receives medical images as inputs. Subsequently, a Deep Convolutional Neural Network (DCNN) is utilised to perform dimension reduction, extracting crucial information from the images. This extracted information is then used to construct a data matrix, which is further converted into a vector. The vector is processed by a fully connected neural network, which subsequently determines the outputs. For instance, it can make predictions or classify the images. Machine learning algorithms can analyse large amounts of patient data, such as medical images, genetic information, and medical records, to identify patterns and make accurate determinations about the presence of disease or something entirely new.

Photo from Serdar Abut, Hayrettin Okut, Rosey Zackula and Ken James Kallail Deep Neural Networks and Applications in Medical Research Published: 13 September 2023
Recent advancement
What ECG can predict ?
As an experienced physician, given an ECG, we are all able to estimate the gender of the patient with 50% accuracy. However, with the use of an AI model, ECG can predict the gender of the patient with an area under the curve (AUC) of 0.97 (7). Ejection fraction can be predicted by ECG with a deep learning model showing an AUC of 0.9472, with a sensitivity of 86.9% and specificity of 89.6% (8). ECG can also predict anaemia (9), AF and stroke (10), diabetes (11), CKD (12), and hyperthyroidism (13).
What CXR can predict ?
CXR in A&E is typically used to determine pulmonary disease. However, with the use of an AI model, CXR can predict a patient's race with an AUC of 0.91-0.99 (14), diabetes (15), and multiple cardiac functions and valvular heart diseases (16). These are entirely new areas that no radiologist or clinician could identify, but the machine did.
What can a retinal photo predict?
Retinal vessels may provide a readily accessible surrogate approach to studying vascular disease. With computer vision, a lot of new things can be identified that were not visible to the human eye. Retinal photos are able to predict diabetes (17), CKD (18), liver and gallbladder disease (19), heart calcium score (20), Alzheimer's disease (21), myocardial infarction (22), and Parkinson's disease (23).
The use of machine learning can process large amounts of data, generate new hypotheses, and provide insights that help clinicians apply novel interpretations of simple investigations. There is still a long road ahead for the integration of machine learning into our daily practice. Further large-scale prospective studies and validation of models will be needed. We hope that the use of AI will assist emergency physicians in diagnosis and help reduce medical errors.
References